Building the Sentient Marketplace: Beyond Dashboards, Towards Real-Time Reflexes
Build a marketplace with reflexes! Explore how event streaming and real-time data create better user experiences and smarter operations.
Is your marketplace data telling you yesterday's news? In a world demanding instant gratification – immediate matches, real-time inventory, dynamic pricing – relying on batch processing is like navigating a Formula 1 race using a rearview mirror. It’s not just slow; it’s dangerous. Many platforms are drowning in data yet starved for actionable, in-the-moment insights. They measure. They report. They react. Late. This isn't just a tech problem; it's an existential threat to user trust and platform viability. We need to build marketplaces with reflexes, not just records. This requires a fundamental shift towards event streaming, truly adaptive interfaces, and pushing intelligence to the edge. Let's dissect how.
Ditch the Rearview Mirror: Why Batch Analytics Kills Marketplaces
The Latency Tax: How Delayed Insights Cost You Users (and Revenue)
Every millisecond counts. That’s not hyperbole; it’s commerce. Users expect instant feedback. A buyer sees an item "in stock" only to find it gone at checkout. A seller waits hours for performance data. A rider waits minutes for a driver match that should be instantaneous. This friction, born from data latency, is the silent killer of user experience. Google research highlights that even a one-second delay in mobile page load times can impact conversion rates by up to 20% (Source: Google/Deloitte Mobile Speed Study). Imagine the impact of similar delays in core marketplace functions. It erodes trust. It drives churn. It’s a direct tax on your revenue.
We saw this firsthand with an e-commerce marketplace client specializing in limited-edition collectibles. Their batch inventory updates, running every 15 minutes, were disastrous during high-demand "drop" events. Users added items to carts simultaneously, exceeding actual stock. The result? Checkout failures, furious customers, and a social media firestorm. The fix wasn't just faster batching; it was rethinking data flow entirely.
The actionable takeaway here is stark: Stop accepting data delays as normal. Your first step? Map your critical user journeys and identify every point where stale data causes friction or failure. Implement basic event tracking for these core interactions immediately. Even simple logging provides more real-time visibility than a daily report.
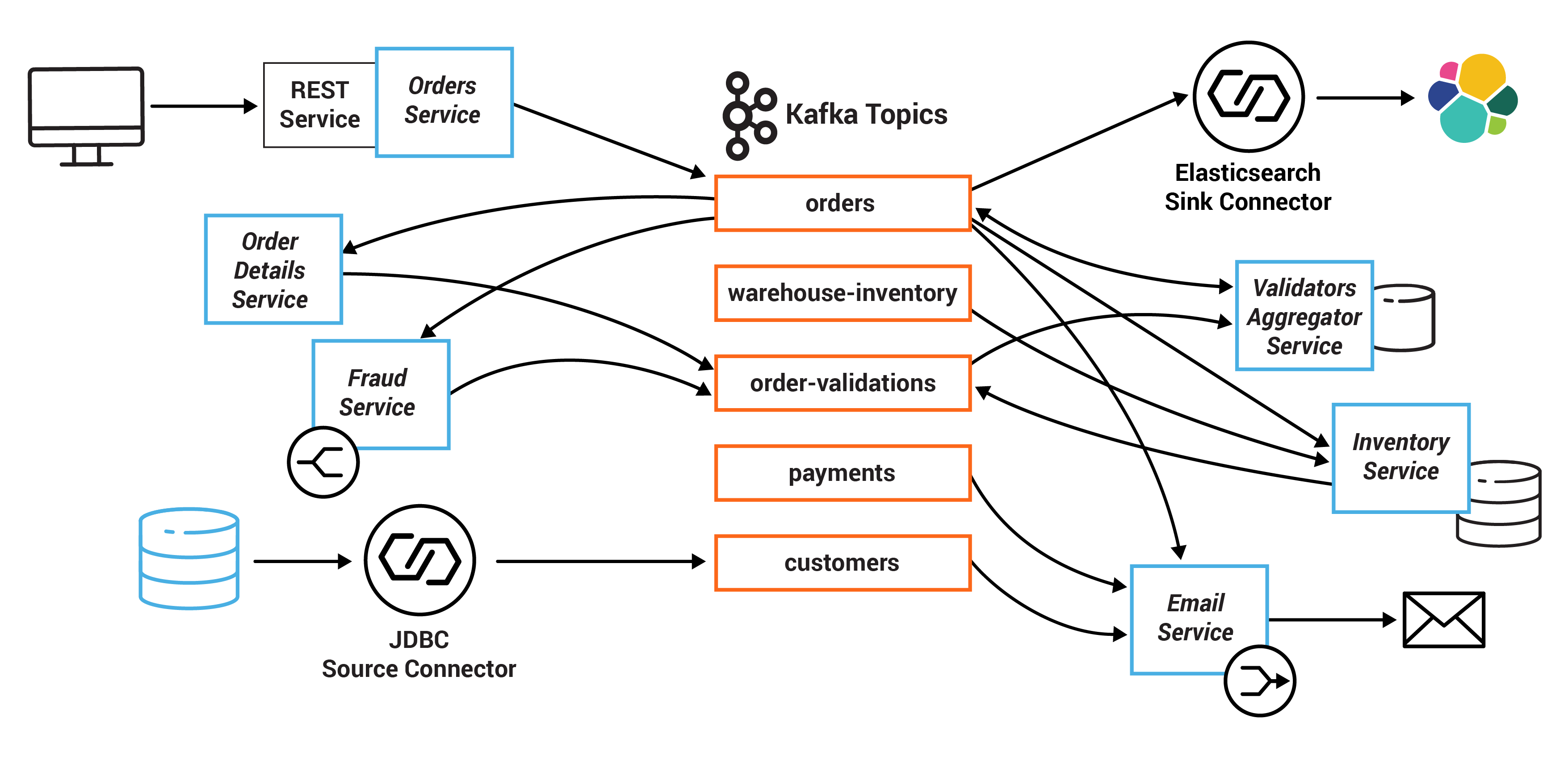
Event Streaming: Your Marketplace's Central Nervous System
Batch processing looks at snapshots. Event streaming watches the movie. Technologies like Apache Kafka or Pulsar aren't just message queues; they are platforms for building a real-time, event-driven architecture. Think of it as your marketplace's central nervous system, processing signals (user clicks, orders, payments, location updates) as they happen. This allows disparate parts of your platform to react instantly and intelligently. Confluent, a major player in the Kafka ecosystem, consistently reports massive adoption, indicating a clear industry shift away from batch (Source: Confluent Data in Motion Reports - general trend reference). This isn't just for tech giants.
Consider a logistics marketplace client 1985 worked with. They struggled with coordinating independent truckers, fluctuating delivery demands, and real-time traffic data. Their legacy systems processed data in silos, leading to inefficient routing and delayed ETAs. By implementing Kafka as a central event hub, we unified GPS streams, order placements, and payment confirmations. This enabled real-time route optimization, dynamic pricing adjustments based on demand, and proactive customer notifications about delays.
The lesson? Start with your core business events. Don't boil the ocean. Define a clear schema for crucial events likeuser_registered
,listing_created
,order_placed
,payment_processed
. The specific streaming platform (Kafka, Pulsar, Kinesis) matters less initially than establishing this event-driven mindset and ensuring data consistency across your services.
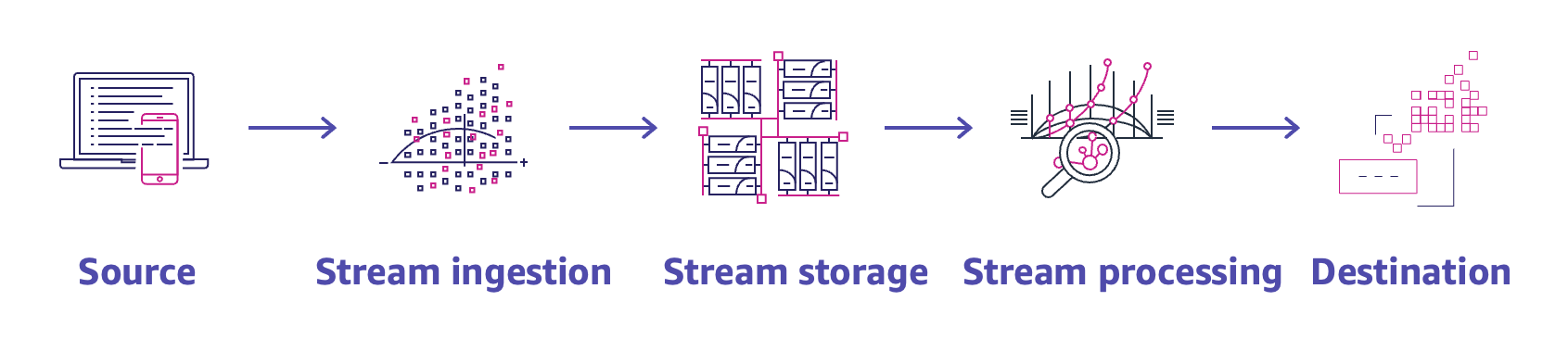
Seeing Clearly: Adaptive Dashboards That Drive Action
Static Dashboards Are Dead: Welcome to Adaptive Intelligence
Your dashboard looks great. Lots of charts. Impressive numbers. But does it change anything? Too many dashboards are digital vanity mirrors – reflecting past performance but offering little guidance for the future. They are static, generic, and often ignored. Gartner frequently points out that a significant percentage of business intelligence projects fail to deliver tangible ROI, often because insights aren't actionable or timely. Static dashboards contribute heavily to this problem. They present data; they don't guide decisions.
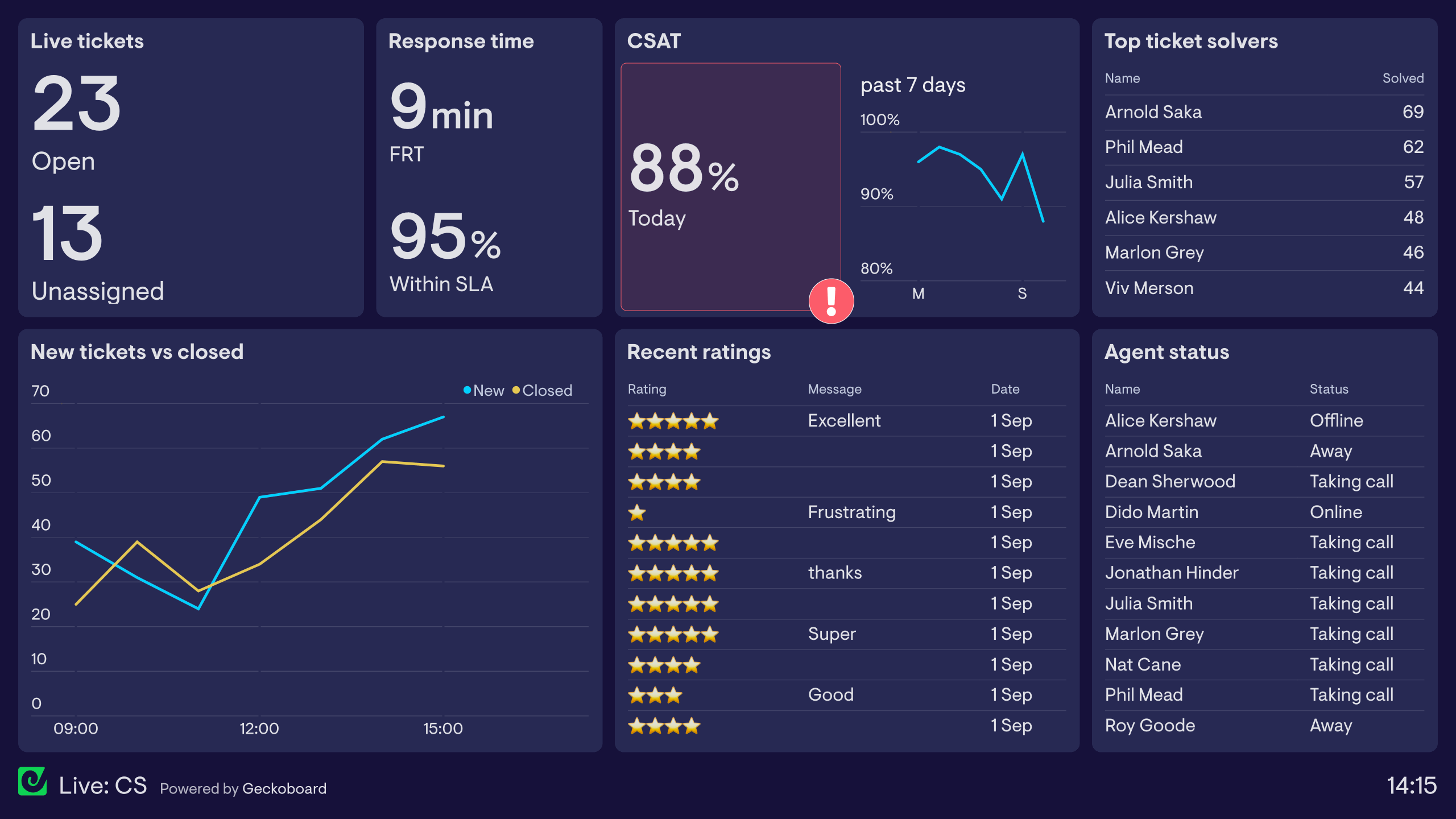
We encountered this with a promising SaaS marketplace founder. They were obsessed with top-line user sign-ups, proudly displayed on their main dashboard. Yet, the business was struggling. Why? Our deep dive, moving beyond their static view, revealed abysmal user activation rates and high churn within the first month. We built a dynamic cohort analysis dashboard that visualized user journeys after sign-up. It wasn't pretty, but it was honest. It showed exactly where users dropped off.
The actionable insight: Focus dashboards on leading indicators, not just lagging ones. Use frameworks like Pirate Metrics (AARRR) but adapt them to your specific user activation flow. What actions predict long-term value? Measure and display those.
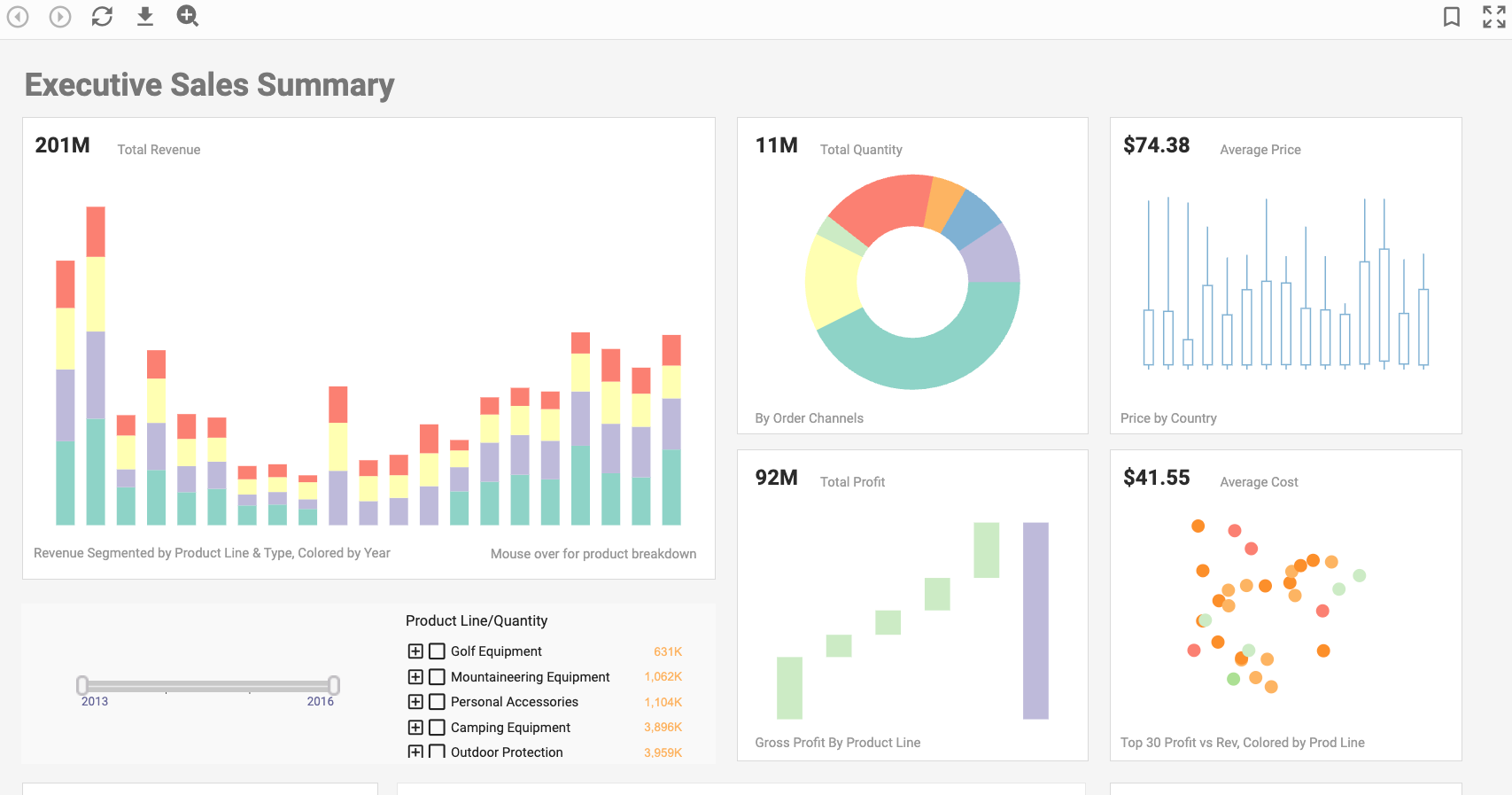
Building Dashboards That Adapt: From Metrics to Decisions
An effective dashboard isn't just a display; it's an interface for action. It should adapt to the user's role, context, and immediate needs. A supplier needs visibility into order fulfillment and inventory levels. A buyer needs personalized recommendations and order tracking. Your operations team needs fraud alerts and platform health metrics. Generic, one-size-fits-all dashboards serve none of them well. Research by Forrester consistently shows that personalized and contextual digital experiences significantly boost engagement and conversion. This applies internally too.
For a B2B equipment rental marketplace, 1985 designed role-based dashboards. Suppliers saw real-time requests matching their inventory, potential maintenance alerts based on usage data (streamed from IoT sensors via events), and yield projections. Buyers saw equipment availability based on location and project needs, with one-click booking. The Ops dashboard didn't just show metrics; it flagged anomalies – unusually long rental durations, payment failures, low-rated supplier interactions – and integrated buttons to trigger investigation workflows or automated communications.
The key takeaway: Design dashboards to trigger action. Embed analytics within operational workflows. Don't force users to switch contexts between seeing an insight and acting on it. Consider alerts, automated tasks, and direct links to relevant management tools.
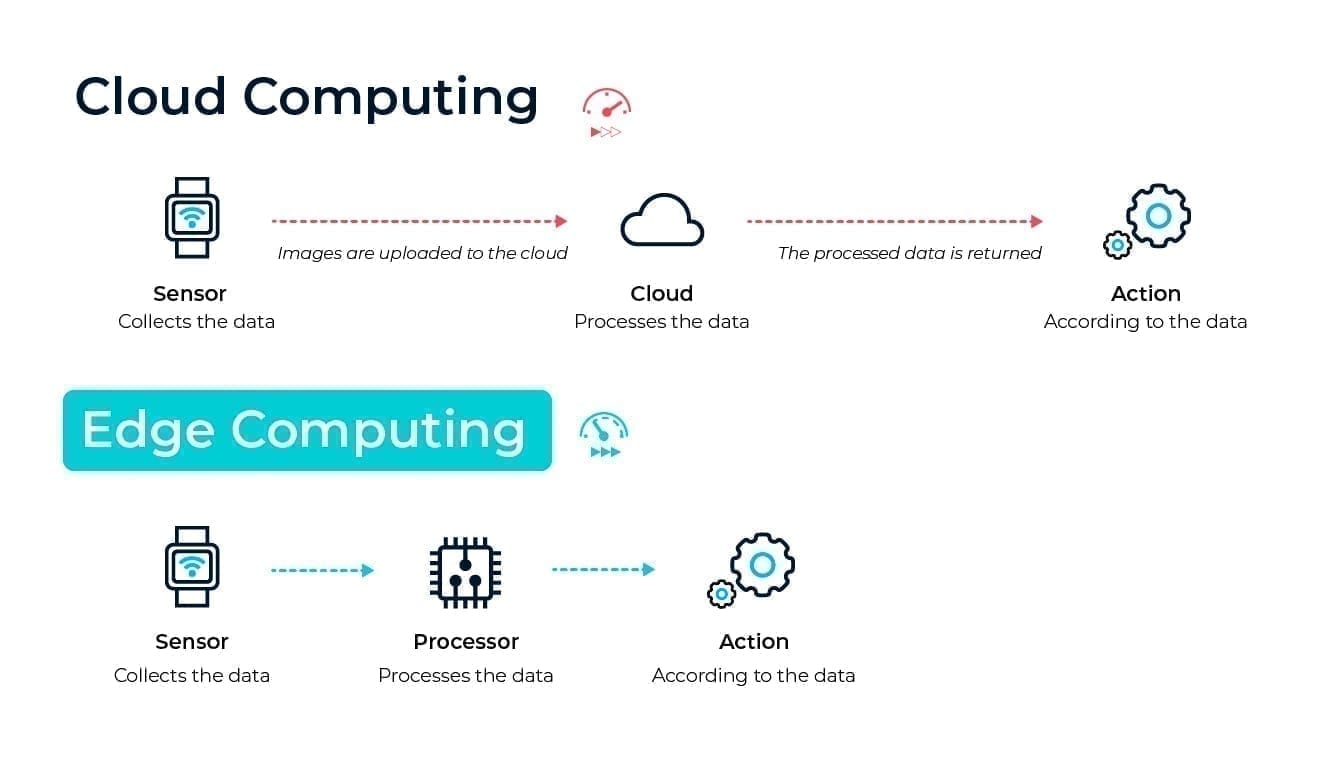
The Edge Advantage: Processing Data Where It Happens
Why Wait? Processing Data at the Source
Sending every click, every sensor reading, every interaction back to a central cloud for processing introduces latency. Sometimes, that delay is unacceptable. Edge analytics involves processing data closer to where it's generated – on the user's device, an IoT sensor, a local gateway, or a point-of-sale system. This minimizes latency, reduces bandwidth costs, and enables real-time responses even with intermittent connectivity. The growth is explosive; IDC forecasts worldwide spending on edge computing to reach hundreds of billions annually, driven by IoT and the need for faster insights.
Think about fraud detection. Waiting for transaction data to hit your central servers before analysis might be too late. A ride-sharing client 1985 partnered with faced issues with GPS spoofing and fraudulent trip completions. We helped implement basic anomaly detection algorithms directly within the driver's mobile app (the edge). The app could flag suspicious location jumps or trip durations inconsistent with distance before the data was even fully synced, allowing for immediate intervention or flagging for review. This reduced reliance on potentially laggy cloud communication and improved the accuracy of real-time earnings calculations.
The lesson: Identify high-frequency, low-latency use cases. Where does instant processing provide a competitive edge or mitigate significant risk? Fraud, critical safety alerts, real-time personalization based on immediate context, and IoT interactions are prime candidates.
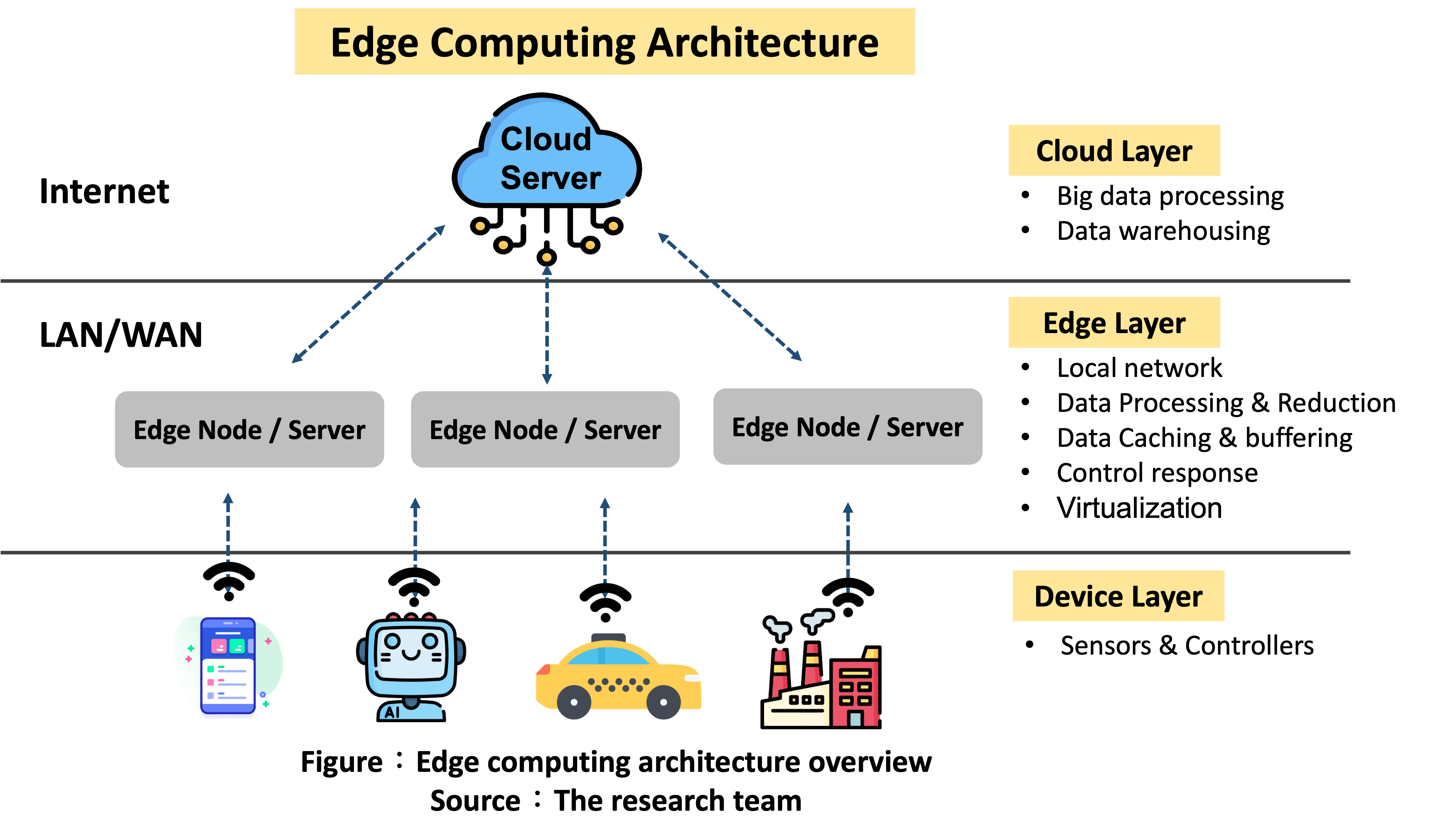
Edge Cases: Real-World Wins
Edge analytics isn't just theoretical; it's delivering tangible results. Frameworks and standards are maturing, making deployment more feasible (e.g., LF Edge initiatives). The applications go beyond simple filtering or aggregation.
We worked with a gig economy platform connecting homeowners with local service providers (plumbers, electricians). A major pain point was verifying task completion accurately and quickly to trigger payments. Relying solely on manual confirmation was slow and prone to disputes. We implemented an edge component in the provider's app that used geofencing to confirm arrival/departure and allowed optional, privacy-preserving image analysis (e.g., confirming a new faucet installation) processed on the device. This data, combined with the customer's confirmation, provided near real-time verification, drastically speeding up payments and reducing support tickets related to job completion disputes.
The takeaway is crucial: Edge isn't limited to complex IoT. Your mobile apps are edge devices. Your partners' POS systems are edge devices. Think creatively about how processing data locally can streamline core marketplace functions. Use an Edge Suitability Checklist: Does this function require sub-second latency? Is the data volume high? Is connectivity potentially unreliable? Does local processing enhance privacy or security?
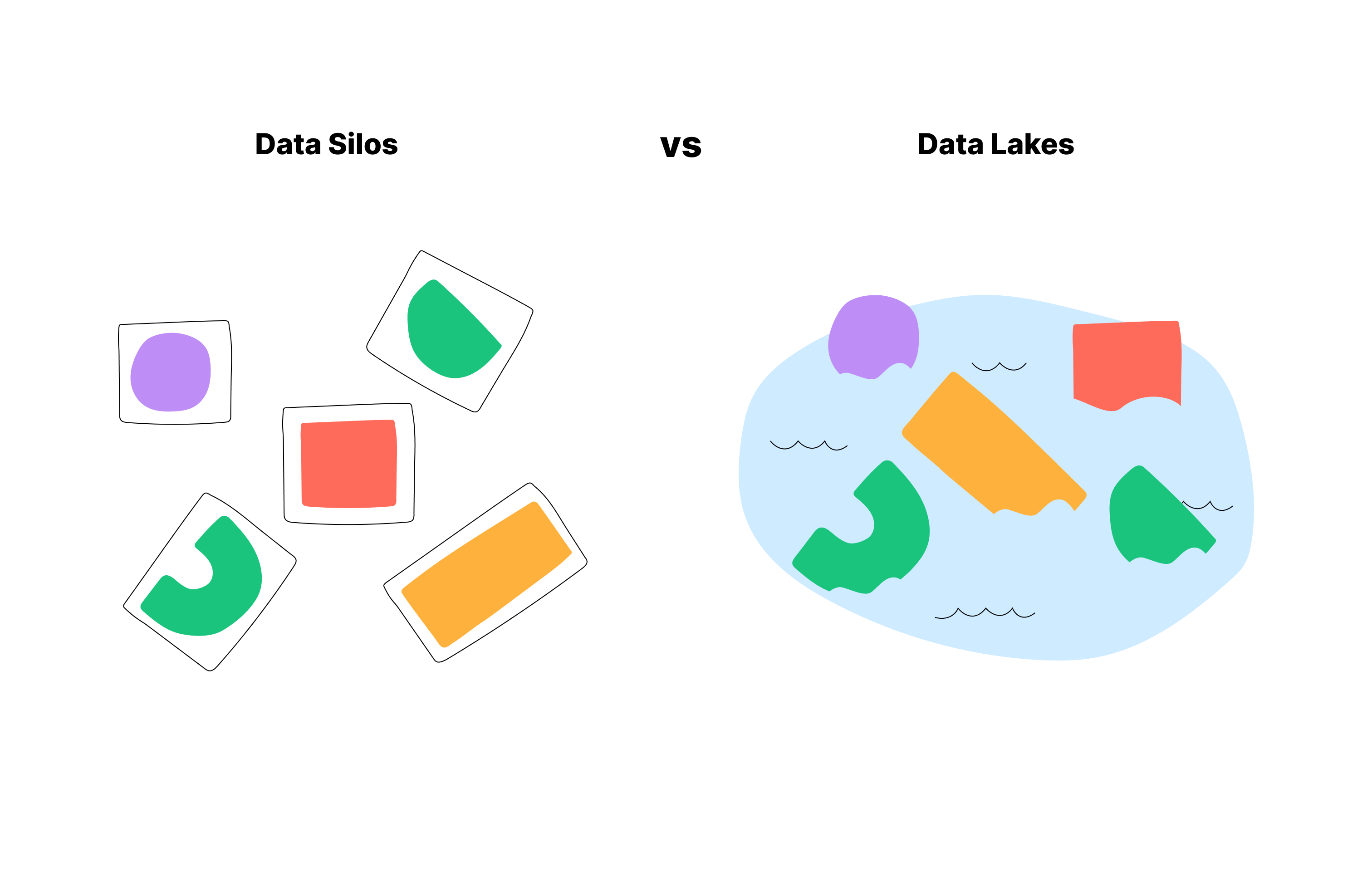
Weaving It Together: The 1985 Approach
From Silos to Synergy: An Integrated Data Strategy
Event streaming, adaptive dashboards, and edge analytics aren't independent tactics; they are interconnected components of a cohesive, real-time data strategy. Implementing them piecemeal without a clear vision leads to fragmented systems and technical debt. The biggest pitfall we see? Teams choosing shiny new tech stacks before clearly defining the business decisions the data needs to enable.
"Our data infrastructure audits frequently uncover expensive, underutilized data lakes or streaming platforms," notes a lead architect at 1985, drawing on internal analysis of client projects. "Often, the core problem wasn't the lack of data, but the inability to surface the right data to the right person at the right time." This resonates with anecdotes from the field. "We burned through nearly $500k building a sophisticated data lake," a fintech CTO confided to us during an initial consultation, "only to realize our immediate, burning need was simply faster, more reliable transaction reporting for regulatory compliance – something a well-designed event stream could have handled more effectively."
The critique is simple: Stop leading with technology; lead with the required business outcome. What decision needs to be made faster or smarter? Work backward from there.
Building for Scale and Sanity
Building a real-time data infrastructure is complex. Doing it monolithically is a recipe for disaster. As the platform scales, tangled data dependencies, slow deployments, and cascading failures become inevitable. While microservices introduce their own coordination challenges, a modular approach to data pipelines is essential for long-term health.
"Our experience across dozens of platform builds shows modular data pipelines, often aligned with microservice boundaries, cut debugging time related to data inconsistencies nearly in half compared to monolithic ETL jobs," reflects 1985's internal best practices documentation. This isn't about chasing trends; it's about resilience and maintainability. One core principle we enforce rigorously is the concept of strict data contracts between services that produce or consume events. Define the schema, version it, and validate it. This prevents the insidious "data drift" – where one team changes a data format without warning, breaking downstream consumers – that quietly cripples scaling marketplaces. It requires discipline, but the payoff in stability and developer velocity is immense.
Here’s a simplified comparison:
Feature | Monolithic Data Pipeline | Modular (Microservice-Aligned) Pipeline |
---|---|---|
Scalability | Difficult; scale entire monolith | Easier; scale individual services/pipelines |
Fault Isolation | Poor; one failure can halt everything | Good; failures typically isolated |
Dev Speed | Slows dramatically with complexity | Faster for independent teams |
Complexity | Initially simpler, grows tangled | Higher initial setup, cleaner long-term |
Maintenance | High risk of breaking changes, hard to debug | Easier to update/debug isolated parts |
Evolve or Evaporate
Building a marketplace today without a robust, real-time data strategy is like launching a ship with holes in the hull. Event streaming gives you awareness. Adaptive dashboards provide intelligent control. Edge analytics delivers instant reflexes. These aren't futuristic nice-to-haves; they are the table stakes for survival and growth in an increasingly demanding digital landscape.
Is your marketplace truly data-driven, or just data-logged? Are your dashboards informing action or just decorating screens? Is latency silently killing your user experience? If your current tech partner isn't proactively discussing event schemas, adaptive UI possibilities, and the strategic use of edge computing, you're likely leaving critical value – and competitive advantage – on the table.
Building a data-first future requires more than just tools; it requires the right expertise and a battle-tested approach. If you suspect your marketplace is flying blind, ping 1985. Let's talk.